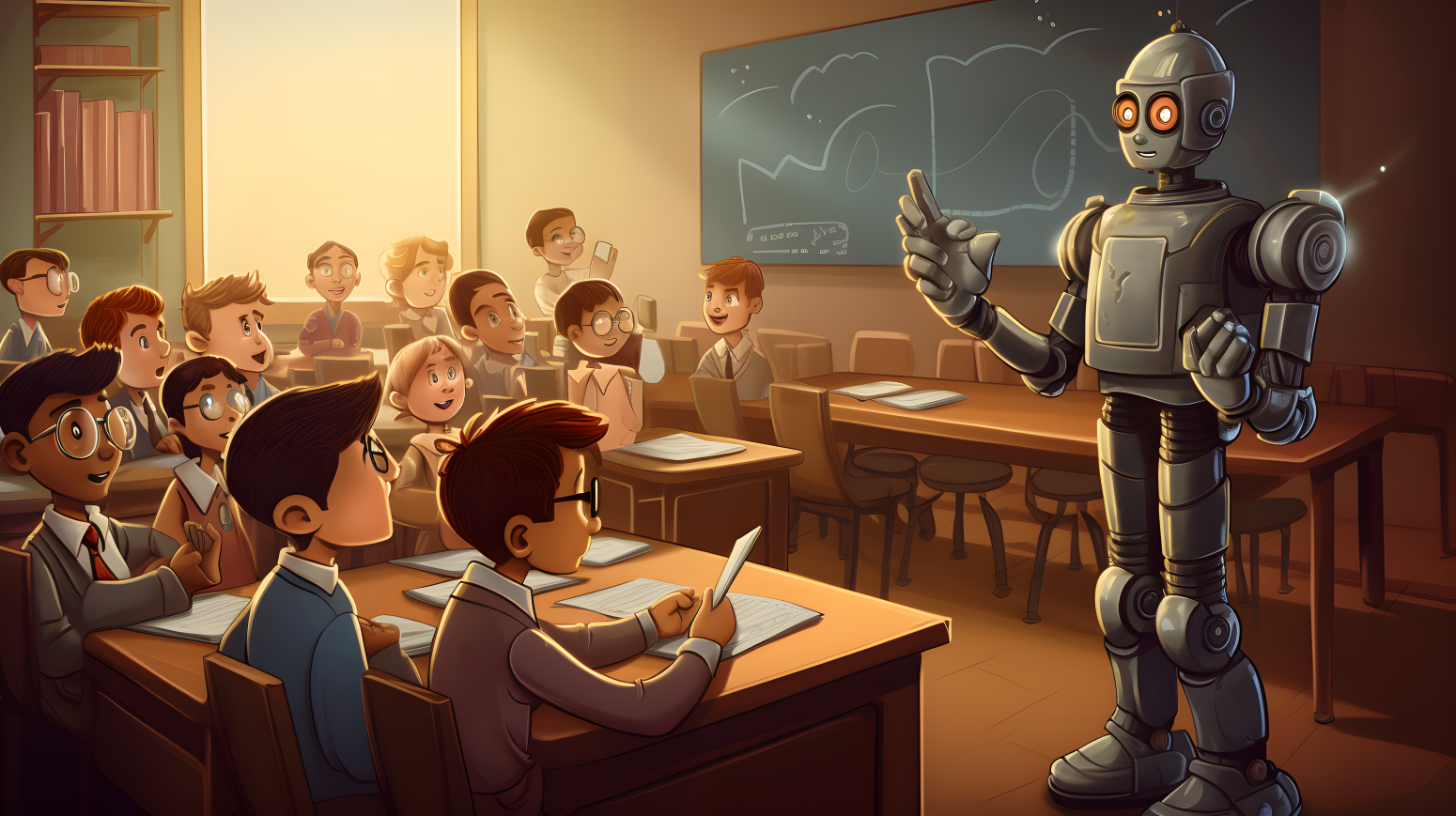
In my last post, I introduced the concept of causal learning and its role in advancing machine intelligence towards human-like capabilities. While conventional machine learning has granted robots with impressive skills — from recognizing complex patterns to adapting in dynamic environments — it has its limitations. As the expectations for robots to operate seamlessly in human-centric environments increase, the demand to understand not just patterns, but the relationships, causes, and logic that drive these patterns, grows exponentially. This is where causal machine learning can play an important role, offering advantages in two areas: generalization and explainability.
A critical challenge in robotics is ensuring that these systems function effectively and safely in unfamiliar, unpredictable, and changing environments. Conventional deep learning approaches, trained on large datasets, often specialize in recognizing patterns within these datasets. Often, when a robot encounters a new situation that is sufficiently different from the training data, performance can dramatically degrade, possibly leading to failure. This is comparable to a student who excels in textbook problems but struggles in real-world applications where the conditions aren’t as neatly defined.
Causal machine learning provides an alternative perspective and focuses on creating a model of the underlying cause-and-effect relationships in data. Rather than strictly recognizing patterns, it seeks to understand the “why” behind these patterns. For a robot, this means understanding the causal relationships between actions and their consequences. With this causal understanding, even if the environment changes, the fundamental relationships often remain consistent, allowing for more robust generalization. A robot that understands the causality of “pushing an object leads to movement” will adapt this knowledge across a variety of surfaces, weights, or object shapes, showcasing a level of adaptability that is often challenging with purely correlative learning.
As robotic systems are introduced into workplaces, homes, and public spaces, the importance of trust in these machines is crucial. Trust is fostered not just by reliable performance but also by understanding the rationale or reasoning of why a robot makes the decisions it does. Conventional deep learning models typically resemble “black boxes,” which might be effective, but their decision-making process is often incomprehensible.
Causal machine learning, with its emphasis on cause-and-effect, inherently leans towards explainability. Decisions made based on causal relationships can be explained in terms of these relationships, offering a clear narrative on why certain actions were taken. For example, if a medical robot decides on a particular intervention, explaining this decision in terms of causal factors (like symptom X typically causes condition Y) is more understandable and trust-building than a vague statement like “this decision matches patterns in previous data.”
As the field of robotics advances, the tools and methodologies that underpin robot cognition and decision-making will play a pivotal role in their success and integration into our lives. Causal machine learning, with its advantages of robust generalization and clear explainability, offers a promising tool to create robots that are not just smart but also adaptable, transparent, and trustworthy.
Image generated with Midjourney